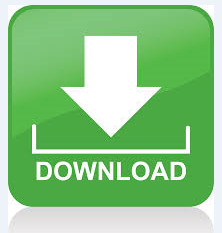
The "principle components" in the data represent the underlying structure in a larger set of measurements that best explain the variation in those measurements. PCA (and other types of dimensionality reduction) is really about seeing the forest for the trees. In this case, that variable might have the straightforward interpretation of "temperature", though in many cases it is not at all clear what the variable represents, or that it even corresponds to a single actual thing in the real world.Īnother way of saying this is that if I know the value of this "latent variable" (the temperature), I can make a pretty good guess at the values of the three observed variables, plus or minus a little noise (the size of which is governed by PC2 and PC3). The fact that there is one principal component that explains most of the variance (i.e., the lengths of PC2 and PC3 are pretty much negligible compared to PC1) suggests that there is a single underlying variable that explains the 3 that we have observed (accidents, pipes, plows). PC2 and PC3 will be vectors sticking out orthogonally to PC1 (see this image, notice how the ellipse is much shorter along blue PC2 and green PC3: ). That is, PC1 (read: the first Principal Component) will point down the tube of the hot dog (think the stick in a corn dog). Essentially, PCA will give us the directions of the main axes of this ellipsoid in order of length. When accidents are low, so are the other two. That is, when accidents are up, so are burst pipes and plow expenses. This suggests that the variables are related, i.e., that they covary. It might happen that the shape of this cloud is very long and thin like a hot dog. We can then draw a tight fitting ellipsoid around these data points to get the general shape of this cloud of points. Now let's fill this space up with a bunch of city-points with their corresponding values. To be explicit, say Montreal had 1000 skidding accidents, had 400 burst pipes, and spent $8000 dollars on snow plows.
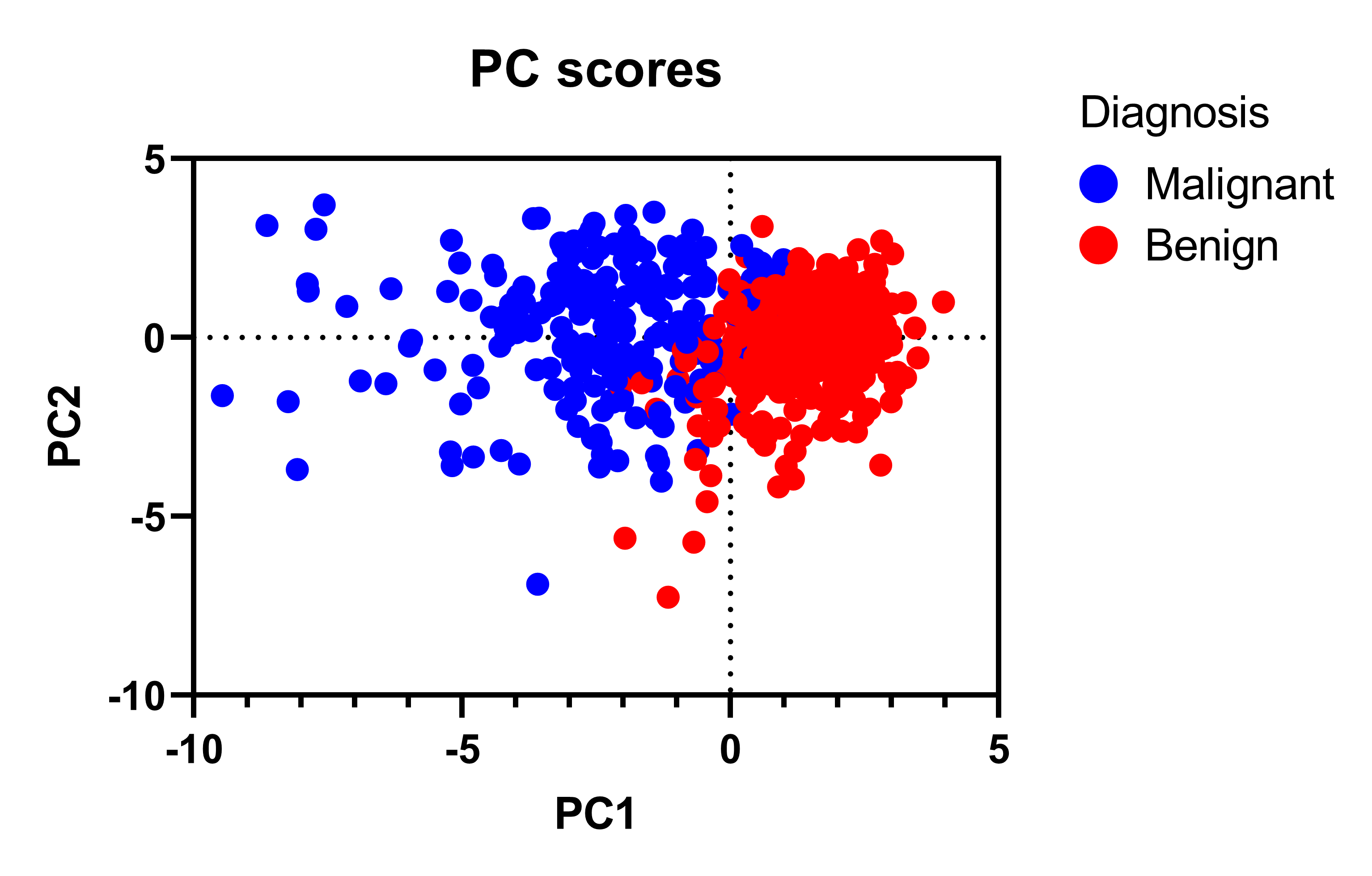
any city we want to consider for which we have this information) can be represented as a point in a 3-dimensional space, where each axis represents one of the variables we are considering. To continue it a little, let's imagine that we only considered the first 3 variables in the data (# accidents, #burst pipes, $ snow plows).
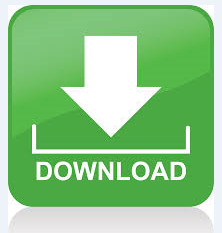